Quick Links
Contact Us
Computer Science DepartmentSwarthmore College
500 College Avenue
Swarthmore, PA 19081
Phone: 610.328.8272
Fax: 610.328.8606
Email: info at cs.swarthmore.edu
Copyright 2009 Swarthmore College. All rights reserved.
Category-Based Intrinsic Motivation
Rachel Lee and Lisa Meeden
Humans process sensory input, evaluate courses of action and adapt to their environments in ways they often take for granted. The goal of this research is to endow robots with a similar ability to learn and adapt. Two key concepts were extracted from current theories of cognitive development: intrinsic motivation and category-formation. Psychologists describe intrinsic motivation as the inherent drive to become familiar with novel stimuli in one's environment. The aim of category-based intrinsic motivation is to develop an open-ended learning system for robots that builds abstractions about an environment and directs learning toward categories where the robot can learn the most. Learning is an increase in the accuracy of predictions about one's perception of the environment. This learning system is tested using a real robot that is allowed to select its own learning experiences, which are ranked by the difficulty of predicting the outcome of a specific course of action. The robot's environment contains an object that moves and an object that does not, elements that show varying degrees of difficulty to learn. By keeping a selective and representative memory of what the robot experiences, the system is able to connect new perceptions to old experiences. It was found that the system's categorization mechanism plays a key role in its overall performance and that, compared to random action selection, this system demonstrates a learning progression. It learns to couple perception with movement in a way that orients it toward the richest learning experiences in its environment.
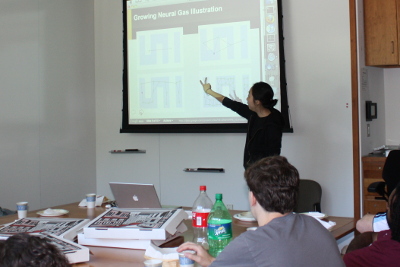